How generative AI allows one architect to reimagine ancient cities
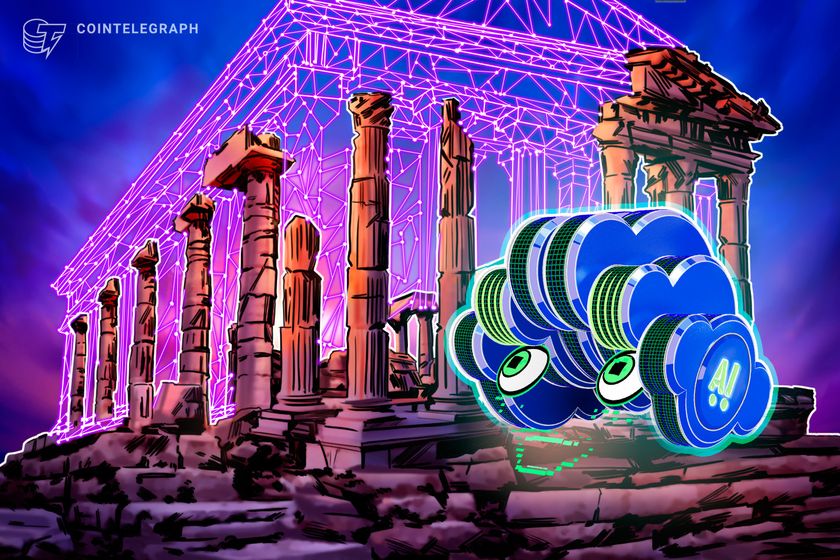
Cointelegraph spoke with architect and designer Tina Marinaki about her work using generative AI and text-to-image prompts to reimagine the ancient Athenian cityscape.
The emergence of generative artificial intelligence (AI) has presented modern society with new means for understanding and visualizing the world.
Meta, the parent company of social media platforms Facebook and Instagram, recently introduced new AI video and image-generating tools for creators, while OpenAI updated the premium version of its popular AI model ChatGPT to include powerful text-to-image generating capabilities.
As the pace of AI development continues to accelerate rapidly, many artists are faced with the challenge of embracing the new tools as a part of their workflow while still managing to keep their unique vision.
One such artist is the New York-based Greek architect Tina Marinaki, who has incorporated AI tools into her creative work and, in the process, created an online community of nearly 20,000 users on Instagram through “Athens Surreal,” which follows her reimagination of the ancient Athenian cityscape.
Cointelegraph spoke with Marinaki about incorporating AI into her work and how she reenvisions her home city using emerging technology.
She explained that the concept of Athens Surreal stemmed from the desire to understand “the way the different AI tools work” while testing ideas for a “different, sometimes romantic, sometimes utopian, futuristic Athens.”
Technical difficulties
According to Marinaki, one of the primary difficulties working with text-to-image AI systems is “translating” an image description to communicate a vision with the AI systems.
“Other challenges are found in algorithmic ethnicity, gender or other biases when algorithms are trained using biased data.”
For example, she reported that a greater number of men can appear in AI-generated images even when a user’s parameters have no mention of gender, and in some cases, AI can create “racist or stereotypical images.”
Despite its biases in text-to-image generation, these weaknesses can lead to strengths if trained correctly.