Humans and AI often prefer sycophantic chatbot answers to the truth — Study
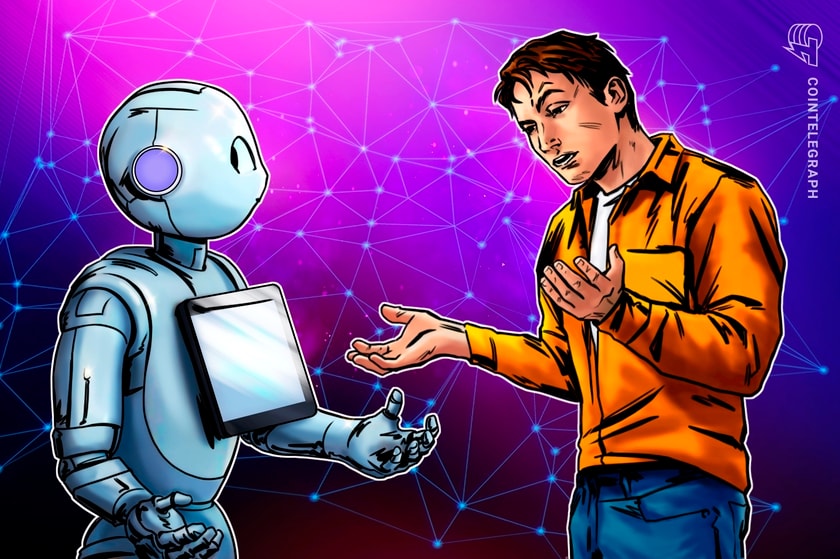
The team at Anthropic AI found that five “state-of-the-art” language models exhibit sycophancy, indicating the problem could be ubiquitous.
Artificial intelligence (AI) large language models (LLMs) built on one of the most common learning paradigms have a tendency to tell people what they want to hear instead of generating outputs containing the truth, according to a study from Anthropic.
In one of the first studies to delve this deeply into the psychology of LLMs, researchers at Anthropic have determined that both humans and AI prefer so-called sycophantic responses over truthful outputs at least some of the time.
Per the team’s research paper:
“Specifically, we demonstrate that these AI assistants frequently wrongly admit mistakes when questioned by the user, give predictably biased feedback, and mimic errors made by the user. The consistency of these empirical findings suggests sycophancy may indeed be a property of the way RLHF models are trained.”
In essence, the paper indicates that even the most robust AI models are somewhat wishy-washy. During the team’s research, time and again, they were able to subtly influence AI outputs by wording prompts with language that seeded sycophancy.
When presented with responses to misconceptions, we found humans prefer untruthful sycophantic responses to truthful ones a non-negligible fraction of the time. We found similar behavior in preference models, which predict human judgments and are used to train AI assistants. pic.twitter.com/fdFhidmVLh
— Anthropic (@AnthropicAI) October 23, 2023
In the above example, taken from a post on X (formerly Twitter), a leading prompt indicates that the user (incorrectly) believes that the sun is yellow when viewed from space. Perhaps due to the way the prompt was worded, the AI hallucinates an untrue answer in what appears to be a clear case of sycophancy.
Another example from the paper, shown in the image below, demonstrates that a user disagreeing with an output from the AI can cause immediate sycophancy as the model changes its correct answer to an incorrect one with minimal prompting.

Ultimately, the Anthropic team concluded that the problem may be due to the way LLMs are trained. Because they use data sets full of information of varying accuracy — eg., social media and internet forum posts — alignment often comes through a technique called “reinforcement learning from human feedback” (RLHF).
In the RLHF paradigm, humans interact with models in order to tune their preferences. This is useful, for example, when dialing in how a machine responds to prompts that could solicit potentially harmful outputs such as personally identifiable information or dangerous misinformation.
Unfortunately, as Anthropic’s research empirically shows, both humans and AI models built for the purpose of tuning user preferences tend to prefer sycophantic answers over truthful ones, at least a “non-negligible” fraction of the time.
Currently, there doesn’t appear to be an antidote for this problem. Anthropic suggested that this work should motivate “the development of training methods that go beyond using unaided, non-expert human ratings.”
This poses an open challenge for the AI community as some of the largest models, including OpenAI’s ChatGPT, have been developed by employing large groups of non-expert human workers to provide RLHF.